Your Enterprise Has to Have AI-Ready Data
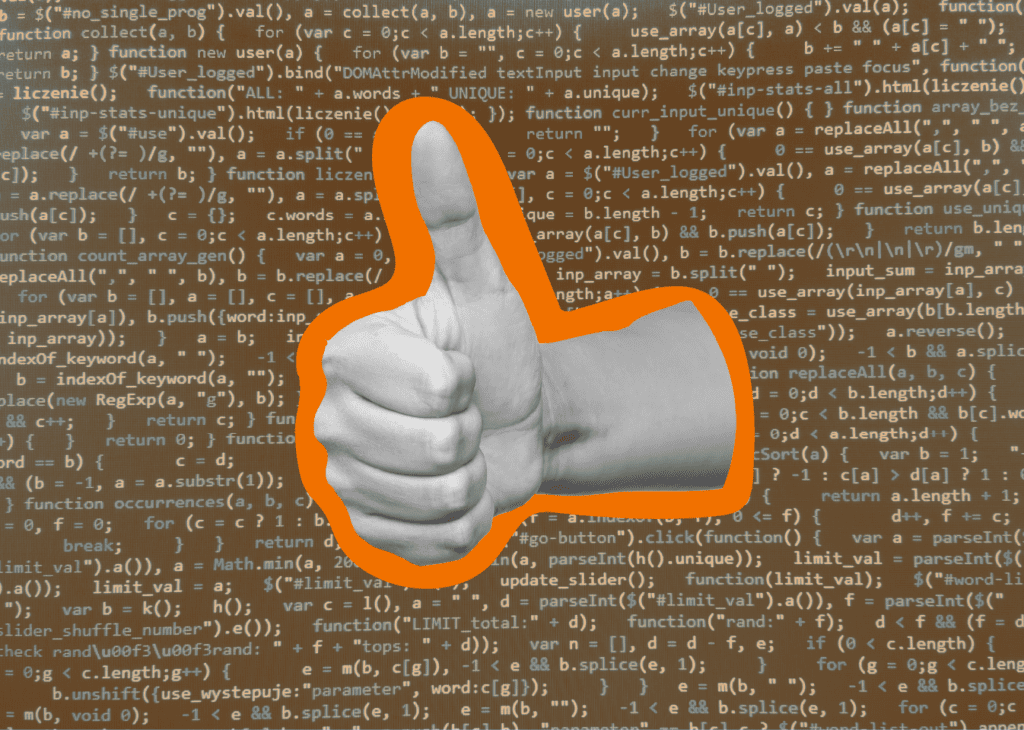
AI is only as good as the data it’s trained on.
Making that data AI-ready isn’t just a technical problem for software engineers. Tech leaders have to make some critical decisions about the data they’re using if they want their AI product or service to be good at doing what they want it to do.
Otherwise, they’ll fail — Forbes reports that as many as 80% of all “AI projects” fail. What separates the successful ones from the rest? They were treated as data projects first.
Forbes contributor Ron Schmelzer writes, “It might seem somewhat obvious to many that AI projects are data projects, but perhaps the AI failures need to understand this at a greater level of detail. What makes an AI system work isn’t specific code, but rather the data.”
Here are some of the mistakes the 80% make (and how you can avoid them).
Mistake #1: Not having a clear data strategy
A data strategy is a comprehensive plan that outlines how an organization collects, manages, and uses data. Too many CEOs, CIOs, and other high-level leaders leave decisions about data entirely to their technical teams. The result is a disconnect between what the business needs and what your AI product or service delivers.
A co-sponsored survey from PwC and Iron Mountain uncovered that “75 percent of business leaders from companies of all sizes, locations and sectors feel they’re ‘making the most of their information assets,’ in reality, only 4 percent are set up for success. Overall, 43 percent of companies surveyed ‘obtain little tangible benefit from their information,’ while 23 percent ‘derive no benefit whatsoever.'”
Be intentional. Start by asking yourself: “How do we use data to get the business outcomes we want?” Again, it seems obvious, but so many organizations aren’t doing it, or if they are, they aren’t effectively communicating the answers.
Mistake #2: Thinking more data is always better
A lot of organizations fall into the “big data” trap. The assumption is that if you collect massive amounts of data, your AI will figure it out.
But quantity doesn’t equal quality here.
Feeding your AI noisy, incomplete, or biased data won’t make it smarter — it’ll make it unreliable. Gartner estimates that poor data quality costs organizations an average of $12.9 million annually. And in AI, those costs grow exponentially because bad data leads to bad decisions at scale.
Collecting less data but ensuring it’s accurate, complete, and correctly formatted will save you time, money, and headaches down the road.
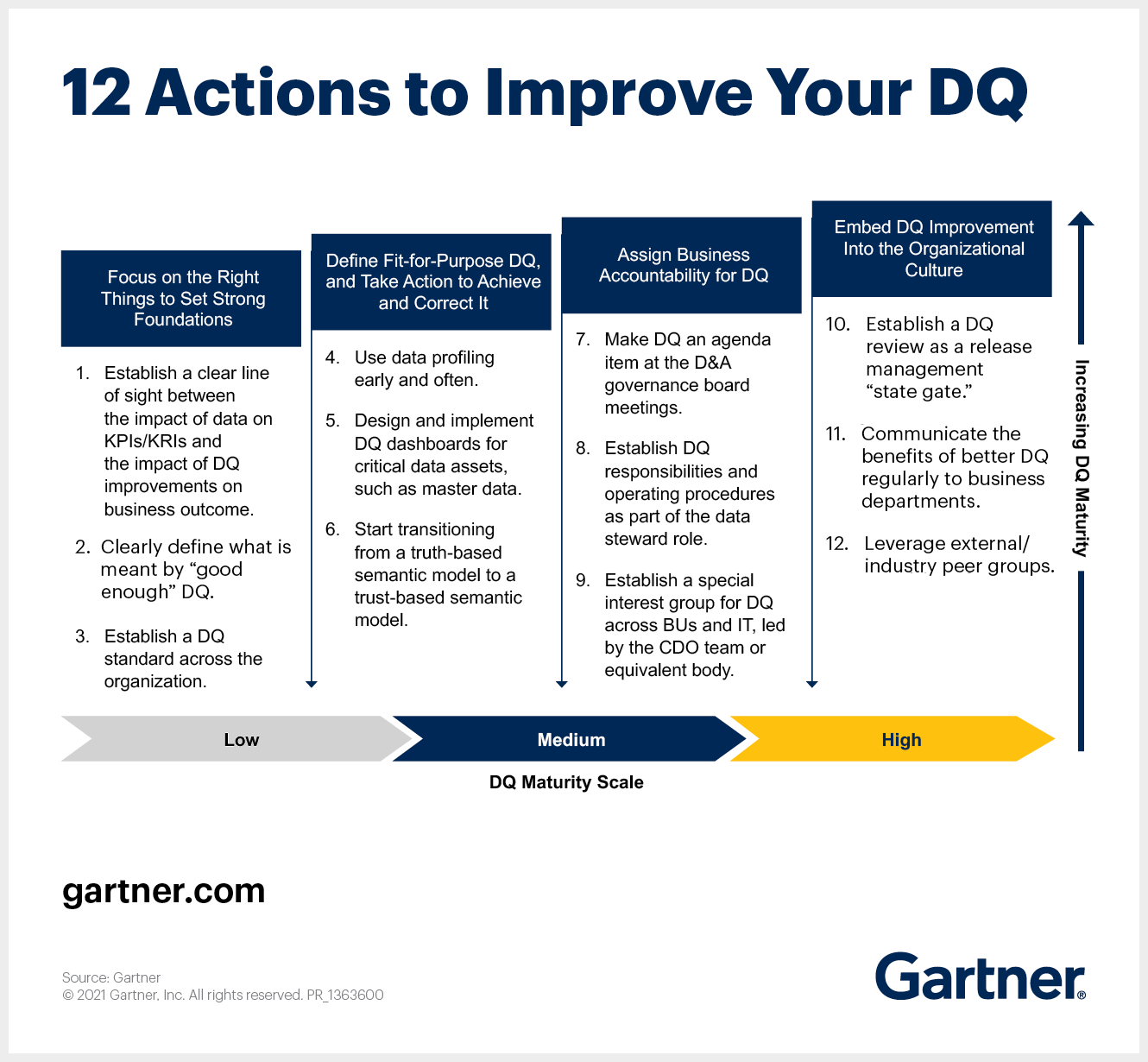
Mistake #3: Treating “data privacy” as a buzzword
Data privacy isn’t just a regulatory box to check. According to Cisco, enterprises earn benefits worth almost 2x their up-front privacy investments.
Why? Because customers trust them more, and trust drives engagement (and quicker sales cycles).
Understand how your data is being used both internally and externally. Kaiser Permanente used industry-standard tracking pixels for some of their applications… and it led to the leak of 13 million people’s data to third parties. Why didn’t anyone know what would happen? How did they not know it was happening? It was a colossal failure of data governance on multiple levels.
If you really want to take data privacy seriously, start questioning your processes. Who has access to data? Are you sharing data with third parties? Do you even have to?
Data first, AI second
You can’t build good AI products without good data, and you can’t have good data unless you have a coherent data strategy that everyone in the organization understands.
AI has the potential to transform whatever you want it to — but only if you have AI-ready data to support it.